On-premises
On-premises infrastructure and software are operated by the user. The term is often used in opposition to on-demand and cloud computing. The user incurs the expenses necessary for the maintenance and operation of software and hardware. He has full responsibility for the operation of the software and is responsible for maintenance. An important advantage of this usage model is that the licensee retains full control over all of their data and the operation of the software.
As part of an infrastructure, a company can own or lease all or part of a data center. For software, it is installed and operated by the user, whether the servers are physical or virtual and whether they are hosted in their data center or in the cloud.
Typical users of the on-premises model are businesses and organizations that collect and process sensitive data that cannot or should not be outsourced to third-party data centers.
- Learn more
- Wikipedia
Related articles
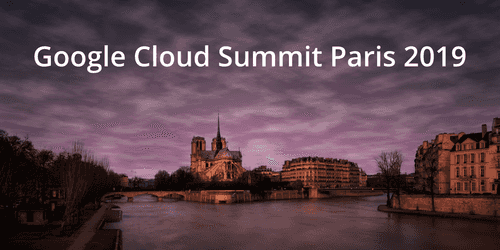
Google Cloud Summit Paris Notes
Categories: Events | Tags: AWS, Azure, Cloud, GCP, Kubernetes, On-premises
Google organized its yearly Summit edition 2019 in Paris on the 18th of June. This yearās event was the biggest yet in Paris, which reflect Googleās commitment to position itself in the French marketā¦
Jun 26, 2019
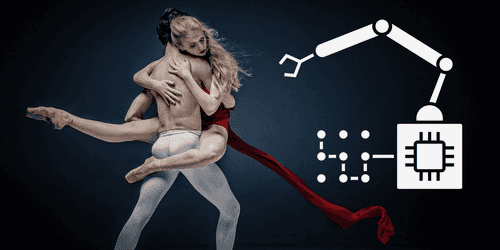
Machine Learning model deployment
Categories: Big Data, Data Engineering, Data Science, DevOps & SRE | Tags: DevOps, Operation, AI, Cloud, Machine Learning, MLOps, On-premises, Schema
āEnterprise Machine Learning requires looking at the big picture [ā¦] from a data engineering and a data platform perspective,ā lectured Justin Norman during the talk on the deployment of Machineā¦
Sep 30, 2019
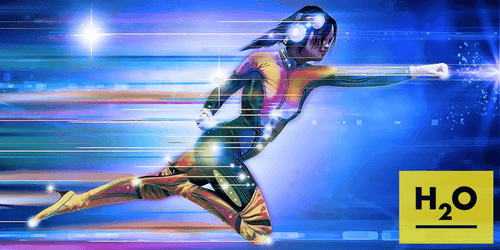
Faster model development with H2O AutoML and Flow
Categories: Data Science, Learning | Tags: Automation, Cloud, H2O, Machine Learning, MLOps, On-premises, Open source, Python
Building Machine Learning (ML) models is a time-consuming process. It requires expertise in statistics, ML algorithms, and programming. On top of that, it also requires the ability to translate aā¦
Dec 10, 2020
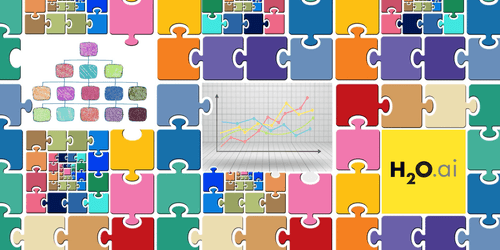
H2O in practice: a Data Scientist feedback
Categories: Data Science, Learning | Tags: Automation, Cloud, H2O, Machine Learning, MLOps, On-premises, Open source, Python
Automated machine learning (AutoML) platforms are gaining popularity and becoming a new important tool in the data scientistsā toolbox. A few months ago, I introduced H2O, an open-source platform forā¦
Sep 29, 2021
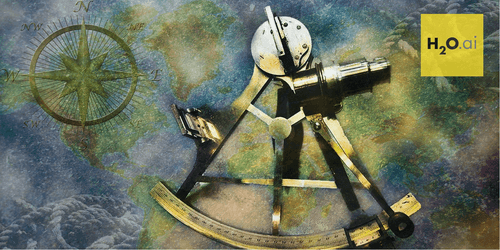
H2O in practice: a protocol combining AutoML with traditional modeling approaches
Categories: Data Science, Learning | Tags: Automation, Cloud, H2O, Machine Learning, MLOps, On-premises, Open source, Python, XGBoost
H20 comes with a lot of functionalities. The second part of the series H2O in practice proposes a protocol to combine AutoML modeling with traditional modeling and optimization approach. The objectiveā¦
Nov 12, 2021