Published articles
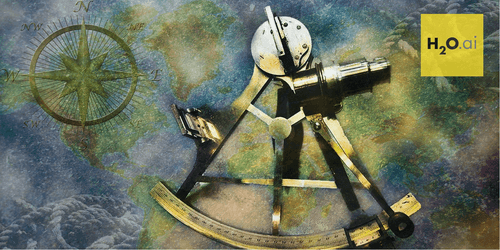
H2O in practice: a protocol combining AutoML with traditional modeling approaches
Categories: Data Science, Learning | Tags: Automation, Cloud, H2O, Machine Learning, MLOps, On-premises, Open source, Python, XGBoost
H20 comes with a lot of functionalities. The second part of the series H2O in practice proposes a protocol to combine AutoML modeling with traditional modeling and optimization approach. The objectiveā¦
Nov 12, 2021
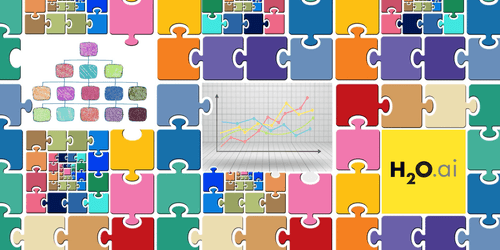
H2O in practice: a Data Scientist feedback
Categories: Data Science, Learning | Tags: Automation, Cloud, H2O, Machine Learning, MLOps, On-premises, Open source, Python
Automated machine learning (AutoML) platforms are gaining popularity and becoming a new important tool in the data scientistsā toolbox. A few months ago, I introduced H2O, an open-source platform forā¦
Sep 29, 2021
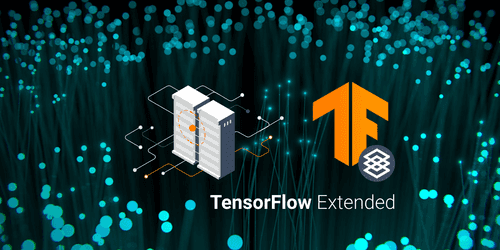
TensorFlow Extended (TFX): the components and their functionalities
Categories: Big Data, Data Engineering, Data Science, Learning | Tags: Beam, Data Engineering, Pipeline, CI/CD, Data Science, Deep Learning, Deployment, Machine Learning, MLOps, Open source, Python, TensorFlow
Putting Machine Learning (ML) and Deep Learning (DL) models in production certainly is a difficult task. It has been recognized as more failure-prone and time consuming than the modeling itself, yetā¦
Mar 5, 2021
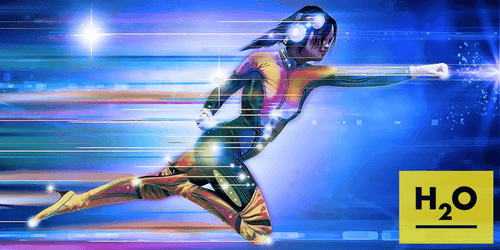
Faster model development with H2O AutoML and Flow
Categories: Data Science, Learning | Tags: Automation, Cloud, H2O, Machine Learning, MLOps, On-premises, Open source, Python
Building Machine Learning (ML) models is a time-consuming process. It requires expertise in statistics, ML algorithms, and programming. On top of that, it also requires the ability to translate aā¦
Dec 10, 2020
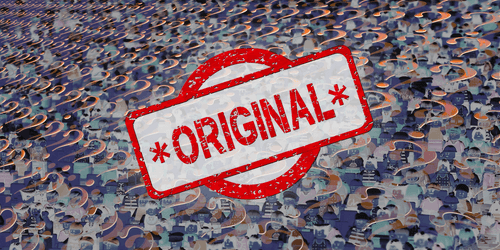
Data versioning and reproducible ML with DVC and MLflow
Categories: Data Science, DevOps & SRE, Events | Tags: Data Engineering, Databricks, Delta Lake, Git, Machine Learning, MLflow, Storage
Our talk on data versioning and reproducible Machine Learning proposed to the Data + AI Summit (formerly known as Spark+AI) is accepted. The summit will take place online the 17-19th Novemberā¦
Sep 30, 2020
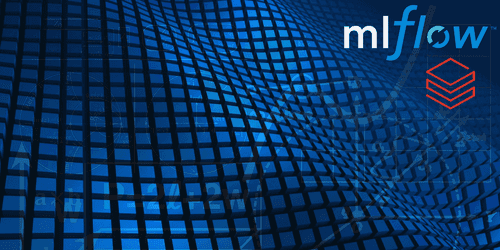
Experiment tracking with MLflow on Databricks Community Edition
Categories: Data Engineering, Data Science, Learning | Tags: Spark, Databricks, Deep Learning, Delta Lake, Machine Learning, MLflow, Notebook, Python, Scikit-learn
Introduction to Databricks Community Edition and MLflow Every day the number of tools helping Data Scientists to build models faster increases. Consequently, the need to manage the results and theā¦
Sep 10, 2020
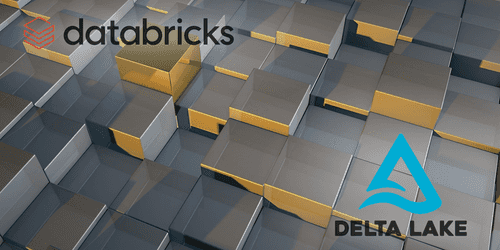
Importing data to Databricks: external tables and Delta Lake
Categories: Data Engineering, Data Science, Learning | Tags: Parquet, AWS, Amazon S3, Azure Data Lake Storage (ADLS), Databricks, Delta Lake, Python
During a Machine Learning project we need to keep track of the training data we are using. This is important for audit purposes and for assessing the performance of the models, developed at a laterā¦
May 21, 2020
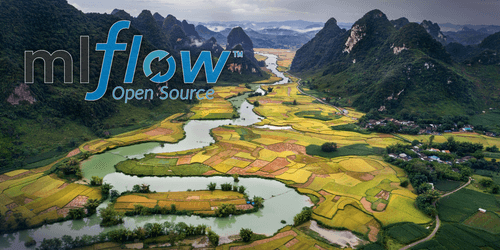
MLflow tutorial: an open source Machine Learning (ML) platform
Categories: Data Engineering, Data Science, Learning | Tags: AWS, Azure, Databricks, Deep Learning, Deployment, Machine Learning, MLflow, MLOps, Python, Scikit-learn
Introduction and principles of MLflow With increasingly cheaper computing power and storage and at the same time increasing data collection in all walks of life, many companies integrated Data Scienceā¦
Mar 23, 2020