Total Cost of Ownership (TCO)
The Total Cost of Ownerwhip is a tool used to estimate the costs of a product or service. It provides a cost basis to determine the economic value of an investment. In opposition to the Whole-life cost, the TCO does not take into the account the early costs (planning, design, construction) nor the later costs (replacement disposal). In the IT industry, the total cost of ownership (TCO) is synonym of the whole-life cost when applied to IT hardware and software acquisitions. The definition evoluated to include all the costs associate with operating a solution or a platform. Such costs not only include the acquisition and operational cost of the product, the platform and services but also the licenses, the speed of treatment, the resilience and the interruption risks, the qualification of new composants and their evolutions, the monitoring, the data sensiblity, the opportunities created by the diversity of the eco-system, the flexibility and the productivity of the teams as well as the Time to Market value. For example, using Erlang as the main programming language can impose a challenge in recruiting or training the engineers to master the language and its ecosystem. However, at the time of its acquision, it allows the Whatsapp team to be constitued of 32 people, of which only 10 worked on the server-side, to serve 450 million active users 2013, to scale the service to 54 billion message on the single day of december 31th 2013 while developping new features, maintaining existing ones and supporting the whole system. Another example is Bleacher Report, a news app and website focusing on sports, which reduce their hardware requirements from 150 servers to 5 when migrating from Ruby to the BEAM platform on which Erlang is running.
- Learn more
- Wikipedia
Related articles
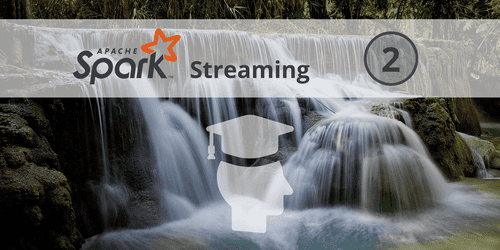
Spark Streaming part 2: run Spark Structured Streaming pipelines in Hadoop
Categories: Data Engineering, Learning | Tags: Spark, Apache Spark Streaming, Python, Streaming
Spark can process streaming data on a multi-node Hadoop cluster relying on HDFS for the storage and YARN for the scheduling of jobs. Thus, Spark Structured Streaming integrates well with Big Dataā¦
May 28, 2019
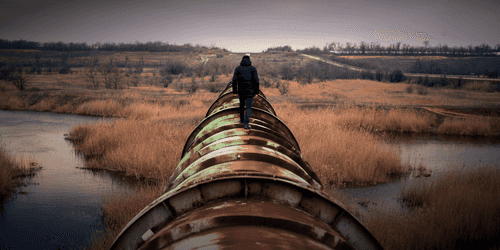
Avoid Bottlenecks in distributed Deep Learning pipelines with Horovod
Categories: Data Science | Tags: GPU, Deep Learning, Horovod, Keras, TensorFlow
The Deep Learning training process can be greatly speed up using a cluster of GPUs. When dealing with huge amounts of data, distributed computing quickly becomes a challenge. A common obstacle whichā¦
Nov 15, 2019
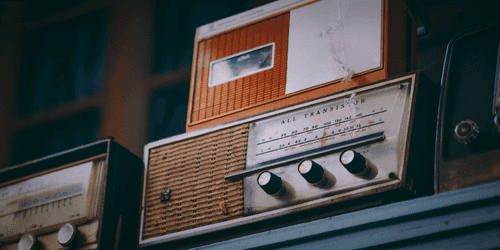
Innovation, project vs product culture in Data Science
Categories: Data Science, Data Governance | Tags: DevOps, Agile, Scrum
Data Science carries the jobs of tomorrow. It is closely linked to the understanding of the business usecases, the behaviors and the insights that will be extracted from existing data. The stakes areā¦
By David WORMS
Oct 8, 2019
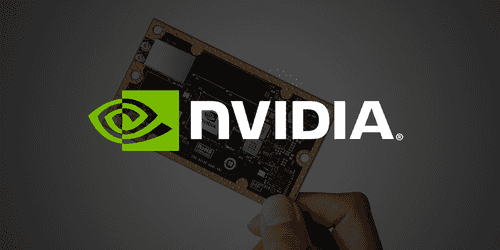
Nvidia and AI on the edge
Categories: Data Science | Tags: Caffe, GPU, NVIDIA, AI, Deep Learning, Edge computing, Keras, PyTorch, TensorFlow
In the last four years, corporations have been investing a lot in AI and particularly in Deep Learning and Edge Computing. While the theory has taken huge steps forward and new algorithms are inventedā¦
By Yliess HATI
Oct 10, 2018
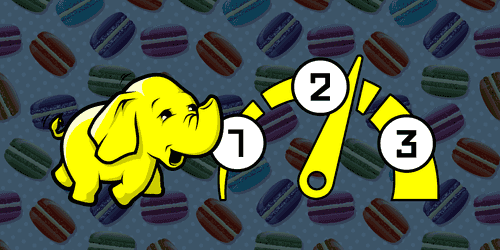
Clusters and workloads migration from Hadoop 2 to Hadoop 3
Categories: Big Data, Infrastructure | Tags: Slider, Erasure Coding, Rolling Upgrade, HDFS, Spark, YARN, Docker
Hadoop 2 to Hadoop 3 migration is a hot subject. How to upgrade your clusters, which features present in the new release may solve current problems and bring new opportunities, how are your currentā¦
Jul 25, 2018
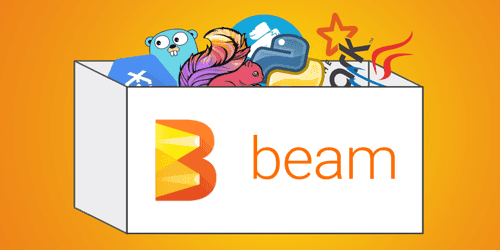
Apache Beam: a unified programming model for data processing pipelines
Categories: Data Engineering, DataWorks Summit 2018 | Tags: Apex, Beam, Pipeline, Flink, Spark
In this article, we will review the concepts, the history and the future of Apache Beam, that may well become the new standard for data processing pipelines definition. At Dataworks Summit 2018 inā¦
May 24, 2018
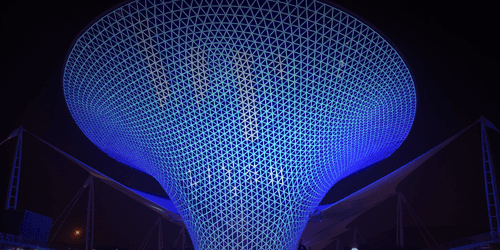
MapReduce introduction
Categories: Big Data | Tags: Java, MapReduce, Big Data, JavaScript
Information systems have more and more data to store and process. Companies like Google, Facebook, Twitter and many others store astronomical amounts of information from their customers and must beā¦
By David WORMS
Jun 26, 2010