Apache ORC
ORC (Optimized Row Columnar) is an open-source column-oriented data storage of the Apache Hadoop ecosystem. It is comparable to Parquet and RCFile, and was created one month prior to Parquet by Hortonworks in collaboration with Facebook. It is highly optimized for reading, writing and processing data in Hive.
ORC files structure includes a Stripe and a Footer.
Stripes: Groups data by chunks.
- Index data: Stored as columns. Keeps min and max values for each column and the row position within each column. It helps locate the stripes and row groups based on the data required.
- Row data: The actual data of the file. Also stored as columns
- Stripe Footer: contains a directory of stream (serialized data) location.
Footer: Collects general file information.
- Metadata: various statistical information related to the columns at stripe level. This enables input split elimination based on predictive push-down which are evaluated for each stripe.
- File footer: contains information of the list of stripes, number of rows per stripe, the data type for each column, and aggregates min, max, and sum at column level.
- Postscript: contains the length of file footer and metadata, the version of the file, the general compression used (none, zlib, snappy, etc), and the size of the compressed folder.
The default stripe size is 250 MB. Large stripe sizes enable large efficient reads from HDFS.
This format includes support for ACID transactions, built-in Indexes, and support for all Hive's types: structs, lists, maps, and unions. It is efficient for Business Intelligence workload and improves performances on read, write and processing in Hive.
Projects using ORC includ Hadoop, Spark, Arrow, Flink, Iceberg, Druid, Gobblin and sdasNifi.
- Learn more
- Official website
Related articles
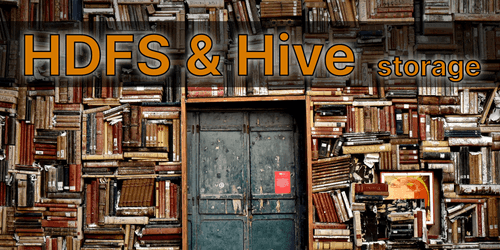
HDFS and Hive storage - comparing file formats and compression methods
Categories: Big Data | Tags: Business intelligence, Hive, ORC, Parquet, File Format
A few days ago, we have conducted a test in order to compare various Hive file formats and compression methods. Among those file formats, some are native to HDFS and apply to all Hadoop users. Theā¦
By David WORMS
Mar 13, 2012
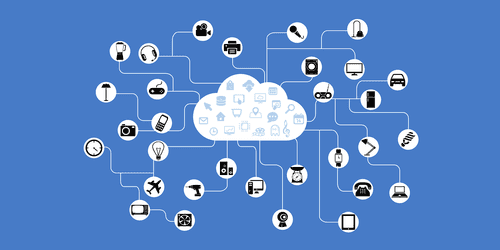
Essential questions about Time Series
Categories: Big Data | Tags: Druid, HBase, Hive, ORC, Data Science, Elasticsearch, Grafana, IOT
Today, the bulk of Big Data is temporal. We see it in the media and among our customers: smart meters, banking transactions, smart factories, connected vehicles ⦠IoT and Big Data go hand in hand. Weā¦
By David WORMS
Mar 18, 2018
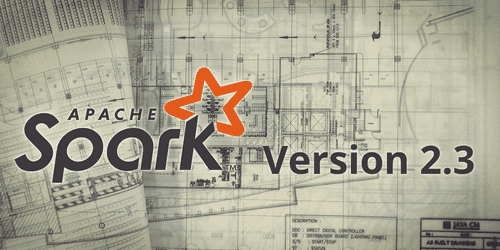
What's new in Apache Spark 2.3?
Categories: Data Engineering, DataWorks Summit 2018 | Tags: Arrow, PySpark, Tuning, ORC, Spark, Spark MLlib, Data Science, Docker, Kubernetes, pandas, Streaming
Letās dive into the new features offered by the 2.3 distribution of Apache Spark. This article is a composition of the following talks seen at the DataWorks Summit 2018 and additional research: Apacheā¦
May 23, 2018
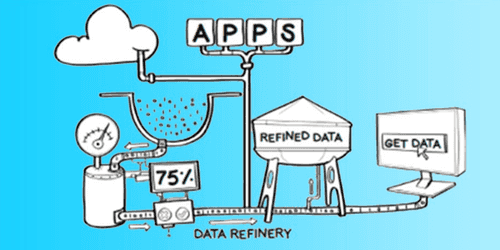
Data Lake ingestion best practices
Categories: Big Data, Data Engineering | Tags: Data Governance, HDF, Operation, Avro, Hive, NiFi, ORC, Spark, Data Lake, File Format, Protocol Buffers, Registry, Schema
Creating a Data Lake requires rigor and experience. Here are some good practices around data ingestion both for batch and stream architectures that we recommend and implement with our customersā¦
By David WORMS
Jun 18, 2018
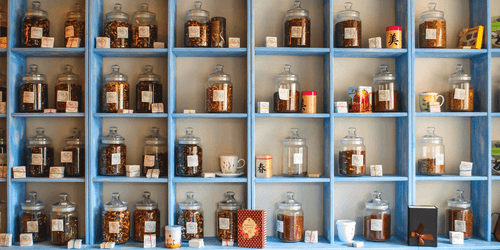
Comparison of different file formats in Big Data
Categories: Big Data, Data Engineering | Tags: Business intelligence, Data structures, Avro, HDFS, ORC, Parquet, Batch processing, Big Data, CSV, JavaScript Object Notation (JSON), Kubernetes, Protocol Buffers
In data processing, there are different types of files formats to store your data sets. Each format has its own pros and cons depending upon the use cases and exists to serve one or several purposesā¦
By Aida NGOM
Jul 23, 2020
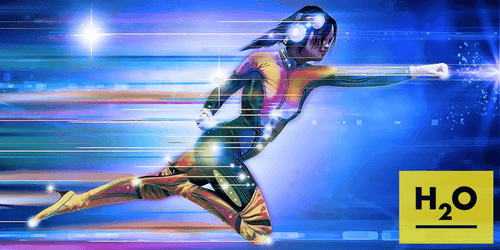
Faster model development with H2O AutoML and Flow
Categories: Data Science, Learning | Tags: Automation, Cloud, H2O, Machine Learning, MLOps, On-premises, Open source, Python
Building Machine Learning (ML) models is a time-consuming process. It requires expertise in statistics, ML algorithms, and programming. On top of that, it also requires the ability to translate aā¦
Dec 10, 2020
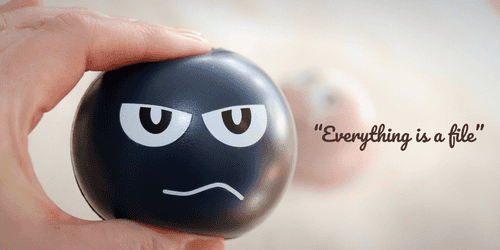
Storage size and generation time in popular file formats
Categories: Data Engineering, Data Science | Tags: Avro, HDFS, Hive, ORC, Parquet, Big Data, Data Lake, File Format, JavaScript Object Notation (JSON)
Choosing an appropriate file format is essential, whether your data transits on the wire or is stored at rest. Each file format comes with its own advantages and disadvantages. We covered them in aā¦
Mar 22, 2021
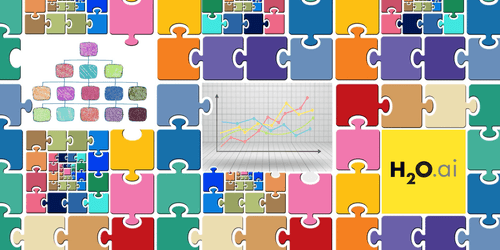
H2O in practice: a Data Scientist feedback
Categories: Data Science, Learning | Tags: Automation, Cloud, H2O, Machine Learning, MLOps, On-premises, Open source, Python
Automated machine learning (AutoML) platforms are gaining popularity and becoming a new important tool in the data scientistsā toolbox. A few months ago, I introduced H2O, an open-source platform forā¦
Sep 29, 2021
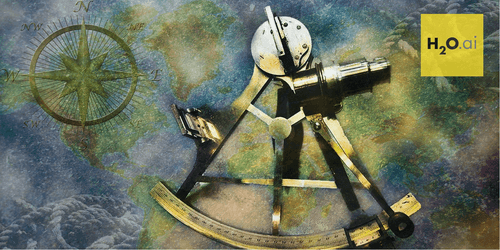
H2O in practice: a protocol combining AutoML with traditional modeling approaches
Categories: Data Science, Learning | Tags: Automation, Cloud, H2O, Machine Learning, MLOps, On-premises, Open source, Python, XGBoost
H20 comes with a lot of functionalities. The second part of the series H2O in practice proposes a protocol to combine AutoML modeling with traditional modeling and optimization approach. The objectiveā¦
Nov 12, 2021
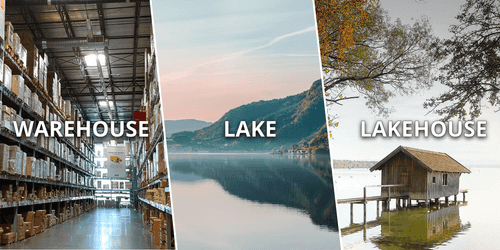
Comparison of database architectures: data warehouse, data lake and data lakehouse
Categories: Big Data, Data Engineering | Tags: Data Governance, Infrastructure, Iceberg, Parquet, Spark, Data Lake, Data lakehouse, Data Warehouse, File Format
Database architectures have experienced constant innovation, evolving with the appearence of new use cases, technical constraints, and requirements. From the three database structures we are comparingā¦
By Gonzalo ETSE
May 17, 2022